Artificial Intelligence (AI) is no longer just a futuristic concept it has become a realistic tool today. With the emergence of conversational AI tools like ChatGPT, Bard, and various other tools there is a growing curiosity about AI and how it can enhance our daily tasks.
For a better understanding of AI’s capabilities, it is essential to grasp its fundamental categories.
AI can be broadly categorized into three types:
1. Strong AI: Strong AI possesses the remarkable ability to come up with solutions for new, unforeseen challenges. This kind of AI can learn and apply knowledge
2. Weak AI: Weak AI operates within a predefined set of instructions designed for specific tasks. It excels at its designated function but cannot extend beyond those boundaries. Common examples include self-driving cars, Siri, and Alexa.
3. Super AI: While still a theoretical concept, super AI is foreseen to surpass human intelligence, capable of solving complex problems beyond our reach.
According to Grandview research, AI is getting more popular with both people and companies in many areas, like research, development, and other fields. Experts estimate it will grow by about 37.3% annually from 2023 to 2030.
In AI, two common types are frequently discussed: predictive AI and generative AI. In this article, we will delve into these two branches, understanding their differences, applications, and the impact they have on various industries.
- Predictive AI vs. Generative AI: The Differences and Applications
- What is Predictive AI?
- How does Predictive AI work?
- Benefits of Predictive AI
- Limitations of Predictive AI
- Applications of Predictive AI
- What is Generative AI?
- How does Generative AI work?
- Performance Measurement of Generative AI
- Benefits of Generative AI
- Drawbacks of Generative AI
- Applications of Generative AI
- Conclusion: Future of AI and its Transformative Power
- FAQs
Predictive AI vs. Generative AI: The Differences and Applications
Predictive AI and Generative AI are two different types of artificial intelligence, each with its unique role in the technological world.
Generative AI is like a digital artist, capable of creating fresh content like images, music, and text. It does this by complex algorithms and learning deeply from its training data.
Predictive AI, however, works differently. It uses statistical algorithms and machine learning to study information and guess what might happen next. It looks at past data to spot patterns and guess what might occur.
Now, where they differ is in their goals. Generative AI models, such as GANs (Generative Adversarial Networks) and language models like GPT-3, are capable of artistic expression and innovation. They can generate creative content like art, music, and text. Generative AI is like a creative artist. But it’s not just for creative stuff that it can help, but also in areas like science and medicine.
Predictive AI is more like a fortune teller. It looks at what has happened before and tries to guess what might happen in the future. It is used in finance to predict how the stock market might go, in healthcare to guess if someone might get sick, and in marketing to figure out what products people might like. It can also be used for creative purposes.
For example, predictive algorithms can recommend personalized content or products to users based on their preferences.
Check this video out: Predictive AI vs. Generative AI | What You Should Know About AI Search
What is Predictive AI?
Predictive AI is also called Predictive Analytics or Machine Learning. It uses statistical algorithms to study data and make predictions about future events or behaviors. Imagine it as a smart robot that looks at old information to figure out what might occur in the future.
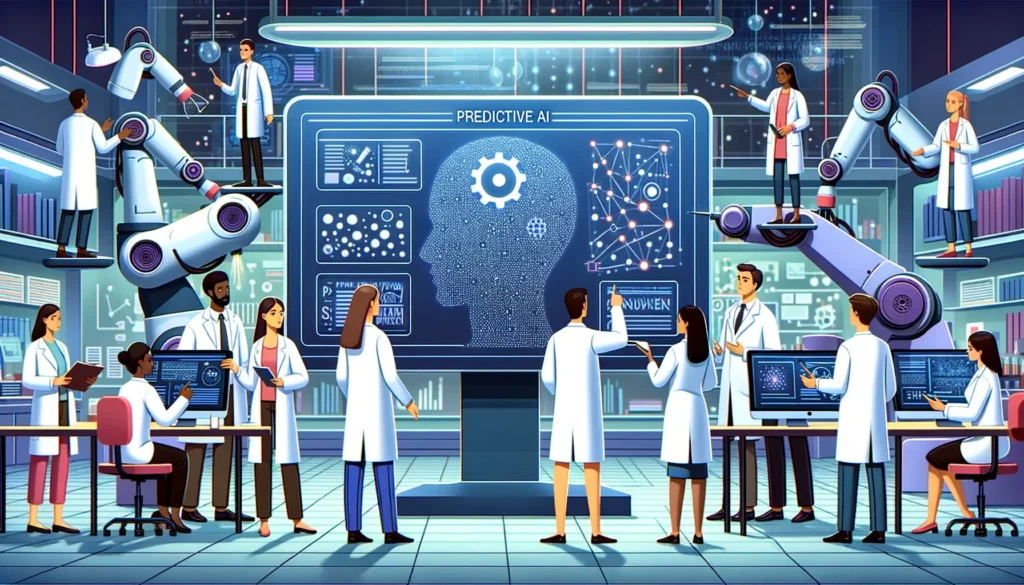
How does Predictive AI work?
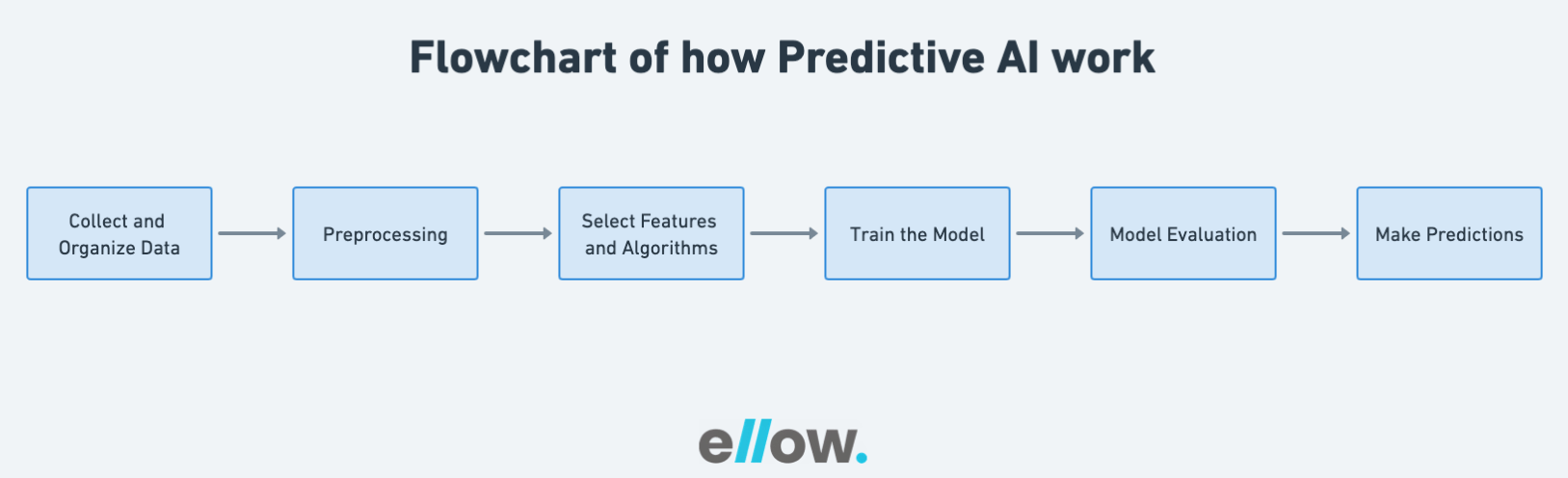
Here’s how it works:
- Collect and Organize Data: The first step is to collect data that is relevant and that has to be analyzed. It is important to make sure that the data collected is suitable and of good quality.
- Preprocessing: Raw data often contains errors or irrelevant information. In this step, the data is cleaned and formatted properly. Any errors or inconsistencies are corrected to ensure that only accurate data is used for predictions.
- Select Features and Algorithms: Choosing the right algorithm is crucial for predictive AI. The accuracy of the predictions depends on the algorithm’s capabilities. Additionally, specific features within the data are selected for the algorithm to focus on. For example, if you want to predict sales, the algorithm might pay attention to factors like past sales data, marketing spending, and economic indicators.
- Train the Model: The selected algorithm is trained using the historical data. This means the algorithm learns from the patterns and relationships within the data. It becomes better at making predictions as it processes more data.
- Model Evaluation: After the algorithm has been trained, its performance is evaluated. This is done by comparing its predictions to known outcomes in the historical data. This step helps determine the accuracy of the model’s predictions.
Benefits of Predictive AI
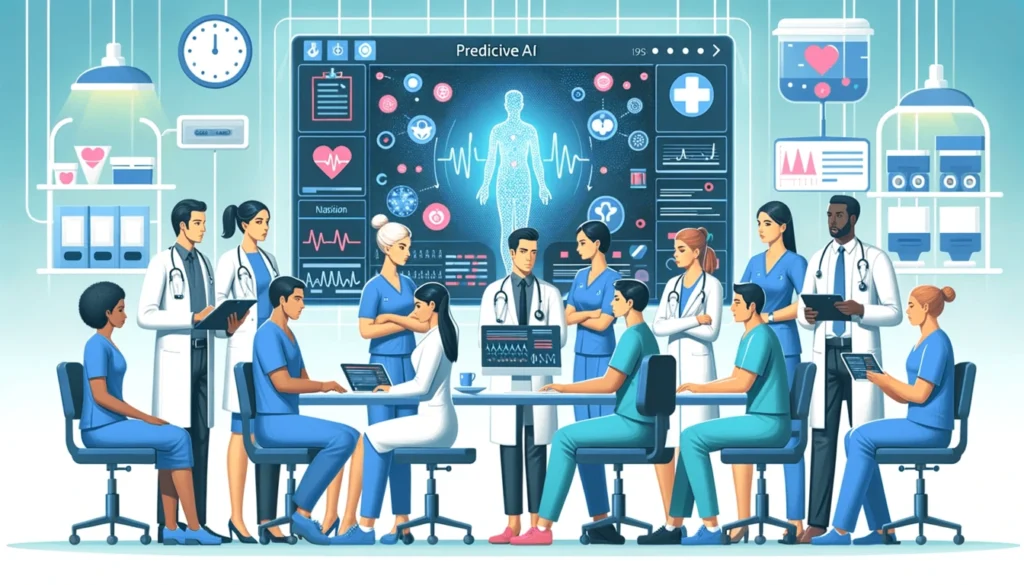
Predictive AI has a lot of benefits that can give businesses a competitive edge, improve decision-making, and enhance efficiency.
1. Competitive advantages
Predictive AI is a powerful tool that allows businesses to stay ahead of their competition. Providing accurate forecasts of future events enables companies to plan strategically and maintain a competitive edge. With the ability to anticipate market trends and customer preferences, businesses can maximize opportunities and stay one step ahead of their rivals.
2. Decision-Making Enhancement:
Predictive AI speeds up the decision-making. In business, having data to support decisions is crucial. Predictive AI helps companies analyze data and simulate different situations to make the best choice using the information they have.
3. Increasing Efficiency:
AI is useful because it can do things very accurately and quickly. When there is a lot of good and accurate data, AI can get the predictions correctly, which means we can do less boring, repetitive work without mistakes. This makes users and businesses work better and faster.
Limitations of Predictive AI
Some of the limitations:
- Data Constraints:
If the data is inconsistent, incomplete, or biased, then the AI can get all its predictions completely wrong. So quality data is important for Predictive AI to work effectively.
- Predictability Challenges:
Predictive AI works well when there are clear patterns in the data. However, not everything in nature follows predictable patterns. In situations where unpredictable events occur or where patterns change over a long period, Predictive AI may generate false patterns and provide unreliable predictions.
- Short Lifespan:
Predictive AI models rely on continuously updating data to make predictions. When data is changing rapidly, the predictions made by the model may have a short lifespan. This means that constant analysis and model updates are necessary to keep the predictions relevant and accurate.
Applications of Predictive AI
Predictive AI finds valuable applications across various fields:
1. Financial Services:
Predictive AI is a major tool in financial services. It helps a lot in detecting financial fraud by identifying unusual data patterns. A bank using Predictive AI to watch the user account. When it sees something unusual, like a big purchase in a place the user has never been, it can quickly alert the bank to check if it is a fraud.
2. Marketing Strategies:
Predictive AI plays an important role in understanding consumer behavior and uplifting marketing strategies. By analyzing past consumer data, it predicts future trends. It helps in giving advertising ideas to businesses by analyzing the past data of the consumer’s purchasing pattern. This kind of targeted approach increases the chances of attracting the right audience and boosting sales.
3. Weather Forecasting:
Predictive AI has transformed weather forecasting. Historical weather data and complex algorithms are used to predict weather patterns more accurately. Using those predictions, the commercial flight industry ensures passenger safety.
What is Generative AI?
Generative AI is a kind of AI that creates data based on the given prompts. This AI uses both machine learning and deep-learning algorithms to come up with new content. Generative AI undergoes a series of dataset feeding and analyzing. The process is as follows:
- Data collection and preparation
- Model architecture selection and initialization
- Model training
- Evaluation and deployment
Unlike predictive AI, which looks at data and makes predictions, generative AI uses what it knows to make new things.
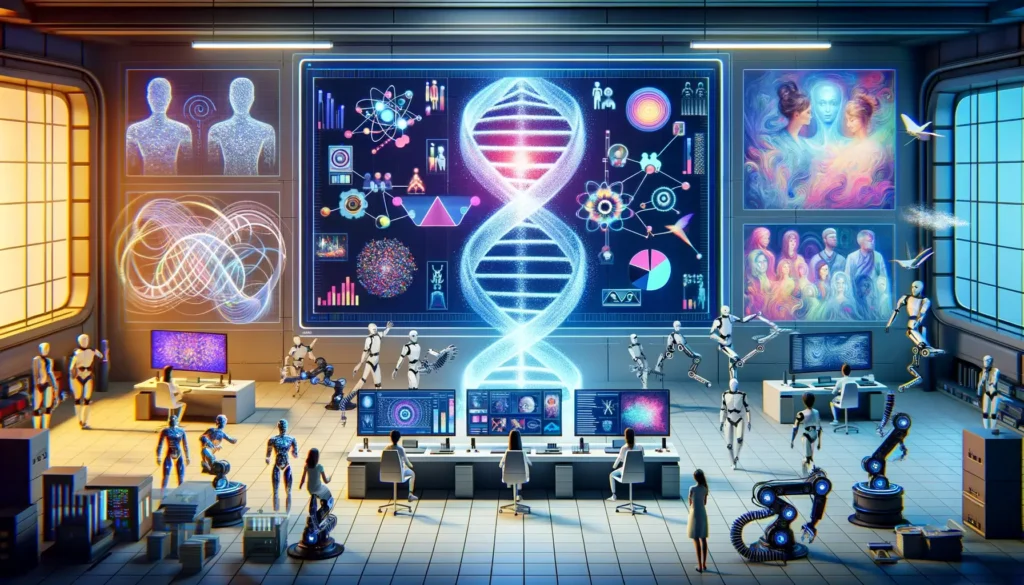
How does Generative AI work?
Generative AI is a smart learner. It has different types of learning, such as learning without a teacher (unsupervised) and learning with a little guidance (semi-supervised), to train itself. This makes the generative AI good at handling lots of data, finding the patterns, and then using these patterns to make new and realistic data. Here are some of the core algorithms and frameworks that help with this creative process:
- Generative Adversarial Networks (GANs):
GANs are very useful in lots of different areas. There are two parts: one is the generator, and the other is the discriminator. The job of the discriminator is to tell apart created data from the original data. The generator learns how to make data that looks just like the original data.
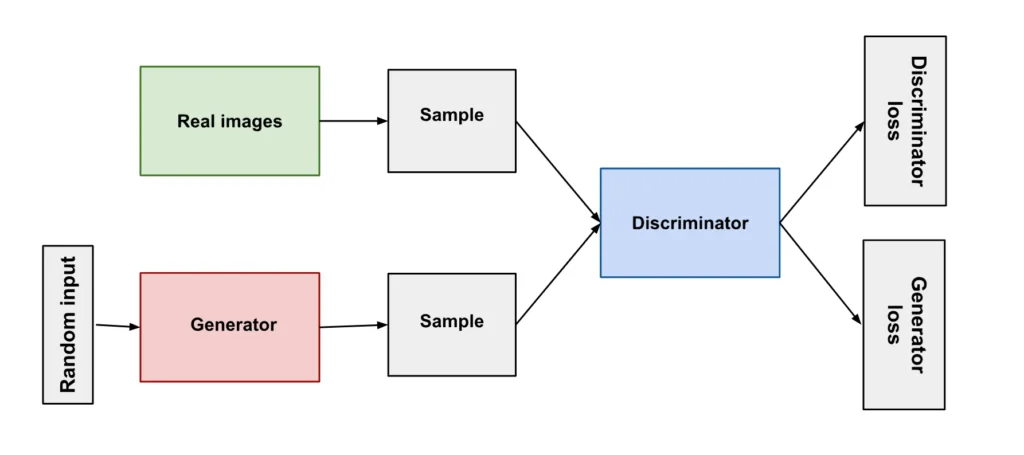
Source
GANs work well for creating data related to a particular topic because they can quickly create good-quality examples with less variation in patterns.
- Variational Autoencoders (VAEs):
VAEs are like creative data magicians. They use a mix of autoencoders (which help simplify data) and some tricks to learn about data. For example, you have pictures of a cat. VAEs can compress these pictures into a particular, compact space where each point represents something about the cat pictures.
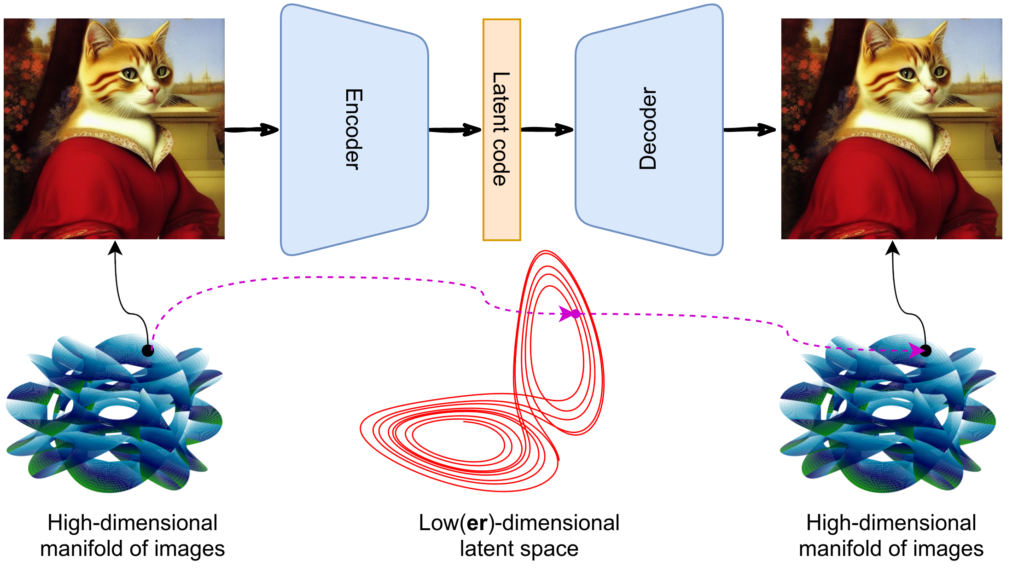
VAEs do it by building two teams. One team (the decoder) tries to turn those points back into cat pictures. The other team (the encoder) takes the regular cat pictures and compresses them into those particular points.
VAEs can use these special points to create brand-new dog pictures that look a lot like the ones they learned from. They can wander around this special space and make all sorts of cat variations. This makes VAEs super creative for making new data that is a lot like the stuff they learned. It is like having a data playground for imaginative research and creating more data.
- Diffusion Models:
Diffusion models or probabilistic diffusion models, are like smart systems that can make data that look similar to the data they were taught. They do this by creating a special kind of path using logic that helps them create the new data.
For example, you have a recipe to make cookies, but there’s a bit of secret sauce added at different steps. This secret sauce is like the magic touch that makes the cookies taste just like the ones you love. This is how diffusion models work. They add Gaussian noise to the data while learning and discovering how to retrieve data via denoising later.
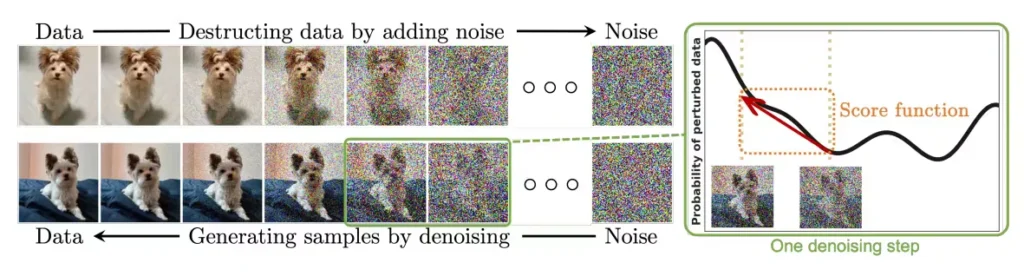
The diffusion model is made up of three important parts: a trained text encoder (like a data translator), a UNet noise predictor (a noise guide), and a variational autoencoder-decoder model (which helps make the final picture look great). These components work together to create a complete and detailed image that’s similar to the ones it learned from.
Performance Measurement of Generative AI
When it comes to measuring how good Generative AI models are, we have to look into a few important things.
1. Accuracy and Quality
An important factor is how accurate and good Generative AI works. For example, if a model is supposed to take pictures from the text (text-to-image generation model) and it makes really bad pictures, then it is not doing a good job and defeats the main aim of the model. The model output should have very close similarities to the real data.
2. Speed and Efficiency
Time is important too. The time needed to train an AI model and the time required by that model to create a realistic output are key performance factors. If the AI takes way longer than a human’s output to create an output, then it’s not very useful. So, the model needs to be fast and not take too much time.
3. Adjustments Required
In addition to speed and time, the amount of changes or fine tunings required before a result is produced is also essential to determine the performance of a model. If the developer has to do a lot of work to create a desired customer expectation using the AI model, then it indicates that the model is not ready for real-world use.
So, to make sure Generative AI models are good, we need to check if the models make good quality content, do they do it quickly, and do they need too many changes to be useful in the real world.
Benefits of Generative AI
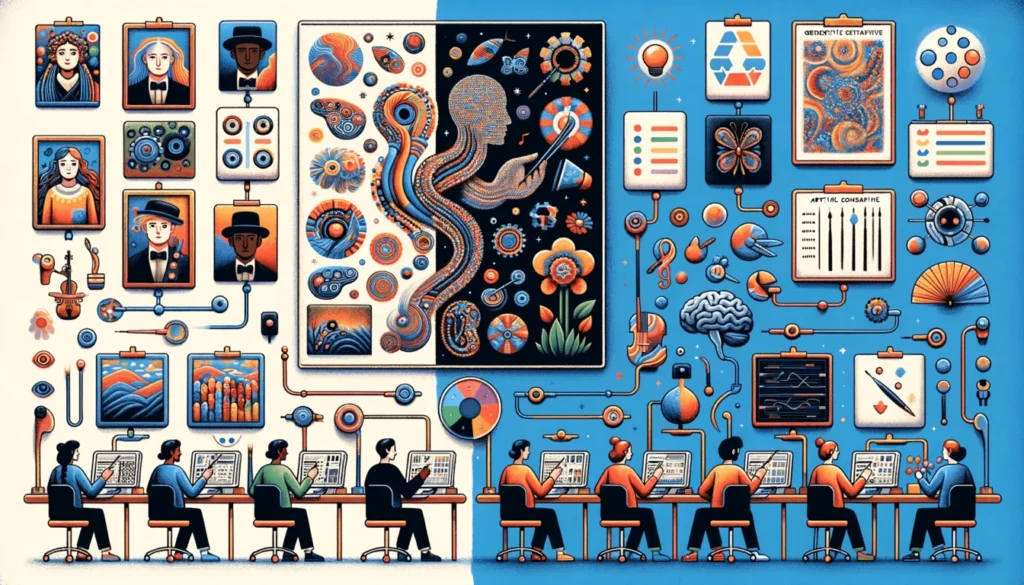
Generative AI offers several advantages that can positively impact various aspects of our work and daily lives. Let’s take a closer look at some of its primary benefits.
1. Increased Efficiency
Generative AI can make tasks faster. For example, it can create content more quickly than humans. This speeds up content creation, helping teams complete more work in less time. Writing articles, designing graphics, or generating code, anything Generative AI can do it quicker.
2. Cost-Effectiveness
Generative AI is cost-effective. It can reduce repetitive tasks, in turn reducing the need for manual labor. This means saving money. Instead of hiring content creators for images, audio, or videos, they can rely on AI. It is like having a skilled workforce without the added costs.
3. Boosted Creativity
Generative AI boosts creativity. It is trained on vast datasets, making it capable of producing creative content. Think about designing unique artwork or composing music. Generative AI can assist or even replace human creativity in these fields, making it a valuable tool for artists and creators.
4. Enhanced Decision-Making
Generative AI plays a role in decision-making. It can simulate scenarios and provide data to fast-track decisions. Imagine a business using AI to model different marketing strategies or product designs. This helps in making decisions quickly, enhancing the business’s ability to serve customers better and improve their overall experience.
Drawbacks of Generative AI
1. Ethical Concerns
Generative AI technology has its drawbacks, including ethical concerns that need careful consideration. There is a potential misuse and dependency on training data. One major ethical concern involves the misuse of generative AI in media creation. This technology can be used to create fake images, videos, and audio content that are almost identical to real ones. For example, fake news articles, misleading videos, and manipulated images can be created using generative AI, leading to confusion and distrust in the media.
2. Dependency on Training Data
The strong dependence of generative AI models on their training data is another big setback. These models do not possess independent reasoning or judgment so they rely entirely on the data they were trained on. If the training data is limited, biased, or inaccurate, it can result in generative AI producing poor content. To mitigate this, it is crucial to provide diverse and ample training data to ensure that the generated content is as unbiased and accurate as possible.
3. Potential for Misuse and Misinformation
In recent times, there has been a rise in the misuse of generative AI for creating misleading and fake content. There are serious implications for privacy, reputation, and the spread of false information. Generative AI’s potential for creating such deceptive content poses a significant challenge that needs to be addressed to maintain trust and integrity in the digital world.
Applications of Generative AI
Generative AI finds various practical applications in today’s world. Let’s explore some of them:
1. Code generation and interpretation:
Generative AI, like ChatGPT, and Google Bard, plays an important role in software development. Developers can use these AIs to make their work easier. Developers can give code snippets or error prompts, and the AI provides solutions for them. Generative AI can also generate sample code based on instructions or prompts given.
2. Chatbots and virtual agents:
Generative AI controls chatbots and virtual agents, making them responsive and efficient. In customer service, chatbots now handle most of the inquiries, reducing the need for human involvement. These AI agents can be trained to answer questions, resolve issues, and provide information quickly.
3. Innovative content generation:
Generative AI helps in creating various forms of content, such as music, videos, images, and more. By analyzing existing samples, it generates new and realistic content. This innovation benefits fields like media, design, and creative arts by streamlining the content creation process. It enables artists and creators to produce fresh and engaging content quickly.
Conclusion: Future of AI and its Transformative Power
In conclusion, the future of AI has immense promise. Generative AI and predictive AI are pivotal players in this technological evolution. Generative AI excels in creating entirely novel content, while predictive AI is skilled at foreseeing future trends. Both models offer substantial benefits across various domains. AI will continue to revolutionize industries like healthcare, finance, and entertainment.
Recommended Read:
AI Trends in Recruitments: Revolutionizing the Hiring Landscape
Top 10 Ways AI Is Revolutionizing Fintech
FAQs
Q1. What is predictive AI?
Predictive AI uses smart technology to look at past information and guess what might happen next. It’s like a crystal ball that helps make educated guesses about the future. People use it in different jobs like finance, healthcare, and marketing to make smarter decisions.
Q2. What are generative AI examples?
Generative AI is all about creating new things. Here are some examples:
1. GPT-3, which writes human-like text.
2. StyleGAN, making realistic pictures.
3. DeepDream, crafting cool patterns.
4. MuseNet, an AI that creates music.
Q3. What’s the difference between generative AI and AI?
Generative AI is a special type of AI. AI covers all smart machines, while generative AI focuses on making new stuff. Other AIs do things like sorting and analyzing data.
Q4. Can generative AI do predictive analytics?
Nope, generative AI’s main job isn’t predicting stuff. It likes to make new things rather than telling the future using old data.
Q5. Can Gen AI be used for prediction?
Generative AI, or Gen AI, is not great at prediction tasks. It’s better at being creative and making new content. To predict things, you’d want to use other AI models designed for that job.